Healthcare big data comprises large datasets generated by various sources. These datasets may consist of both structured and unstructured data in a variety of forms, such as text, images, graphs, or videos. The sheer volume, speed of generation (velocity), and diverse nature (variety) of this data make it “big data”.
This article provides an in-depth discussion of big data in healthcare, its impact on healthcare delivery, its applications, and future implications. The article discusses the transformation of healthcare delivery through big data analytics and innovative healthcare solutions and provides real-world examples and case studies to illustrate the impact of big data in healthcare.
Contents
Understanding big data in healthcare
The advancement of big data analytics in healthcare services has opened the door to novel technologies and the proliferation of healthcare software developers to enhance patient experience and treatment and improve health service delivery. Big data analytics involves integrating and analyzing large amounts of complex heterogeneous data to generate insights, discern patterns, and foster data-driven decision-making in healthcare.
Sources of big data in healthcare range from electronic health records (EHRs) to medical imaging, genomic sequencing, payor records, research data, wearables, and medical devices.
While big data in healthcare promises significant advances, it also presents challenges, such as issues of data privacy and security, and the need for advanced tools and techniques to analyze and manage vast amounts of complex data.
Transformation of healthcare delivery through big data analytics
Big data analytics plays a significant role in transforming the healthcare model from a pay-for-service to a value-based care model. This revolutionary change is possible because big data allows healthcare providers to make decisions driven by extensive data, which can ultimately enhance patient outcomes and lower healthcare costs.
When big data analytics is combined with machine learning, healthcare professionals can generate predictive models that enhance patient care, preempt diseases, and optimize healthcare services. For example, healthcare technology companies like Tempus and Flatiron Health have been leveraging big data to improve cancer research and patient care. Tempus has built the largest library of clinical data to provide medical researchers with a more holistic clinical context to treat each cancer patient individually. On the other hand, Flatiron Health uses large data sets received from patients with cancer to further facilitate research and enhance patient care.
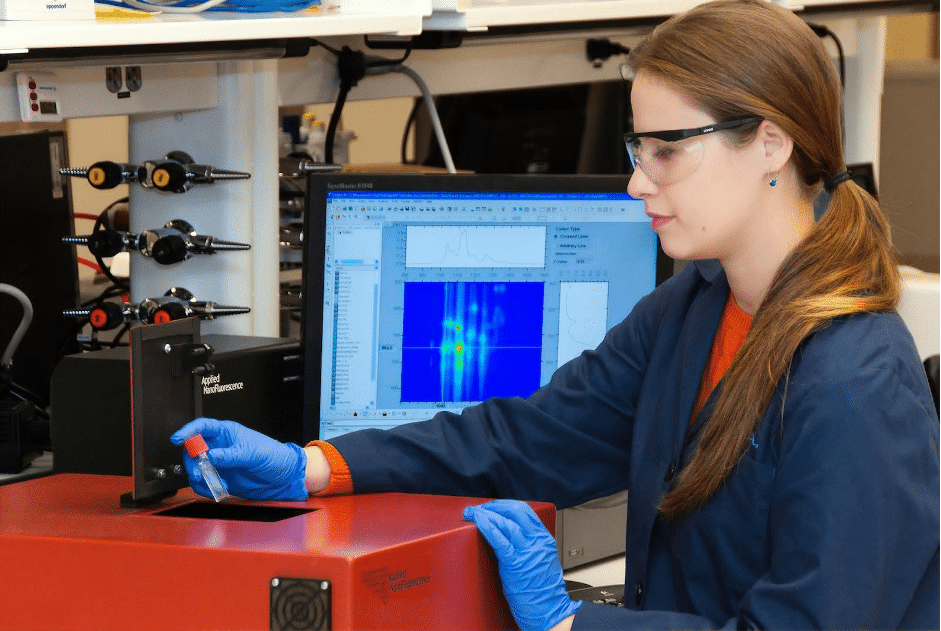
Moreover, the COVID-19 pandemic has significantly highlighted the need for big data analytics in healthcare, prompting innovative approaches to disease tracking, resource allocation, and patient care. KPMG researchers found out that amid the pandemic, the Morehouse School of Medicine in Atlanta developed the National COVID-19 Resiliency Network (NCRN). The NCRN launched breakthrough big data analytics solutions, allowing diverse healthcare organizations across the country to gain access to some valuable information and significantly simplify access to healthcare services.
In addition, big data analysis helps in early disease detection, which is critical for successful treatment. Companies like Pieces and PeraHealth are using big data to improve the early detection of diseases and complications in patients. Pieces is a software and big data analytics company that accumulates data during the whole patient treatment journey to help healthcare practices increase the quality of care. PeraHealth company developed the Rothman Index, which uses data about a patient from various systems to assign a score that reveals whether the patient’s condition is low-, medium-, or high-risk. The scores update in real-time to help clinicians track changes and help patients avoid any health complications.
However, despite the promising potential of big data, its adoption in healthcare isn’t as fast-paced as in other industries due to obvious challenges with securing sensitive patient health data. Nonetheless, with continuous technological improvements, big data is slowly being converted into actionable information that drives the movement toward value-based healthcare, opening the door to remarkable advancements and cost reductions.
Applications of big data analytics in healthcare
Healthcare data analytics applications have seen a remarkable rise in recent years, with the rapid advancement and adoption of technologies like wearable devices and mobile health apps. These technological tools have significantly contributed to the generation of massive health-related data, which when analyzed can provide invaluable insights into patient health, behavior, and healthcare delivery disparities.
Wearable technology in healthcare
The increasing popularity of wearable devices has led to their broad clinical application in healthcare. Wearables can track multiple health-related variables such as physical activity, heart rate variability, nutrition, and mood. The physical activity data from these devices, in particular, has emerged as a robust measure of current health status and future health risks. Moreover, wearables for remote digital health and physical activity monitoring have been validated in diverse settings. Some successful implementations have been seen in digital health programs that incorporate health behavior models and personalized coaching, offering patient-specific measures contributing to the future of precision health.
Nevertheless, challenges persist in the incorporation of physical activity monitoring into routine clinical care due to the need for data standardization among various commercially available devices and integration of this data into EHRs and clinical workflows.
For example, a health organization may use wearable devices to monitor the physical activity of patients with heart disease. The data collected could then be analyzed to understand the relationship between physical activity levels and patient health outcomes, enabling personalized intervention plans to improve the patient’s condition.
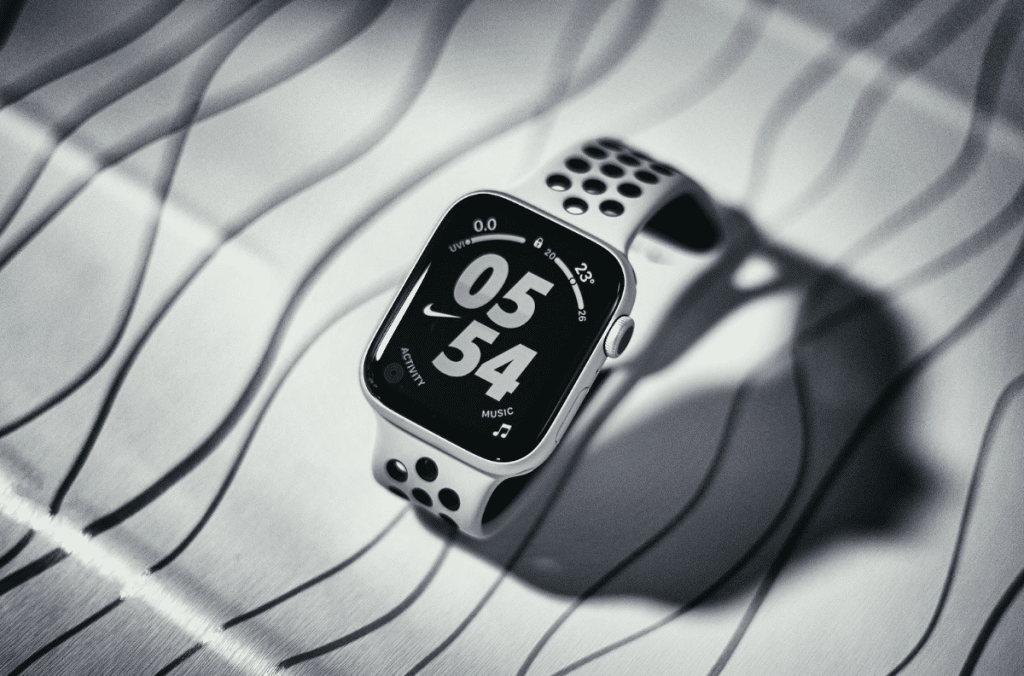
Mobile health apps and smartphone technology
The expansion of mobile health apps and smartphone technology has resulted in a wealth of health-related data. These apps and devices can monitor a wide array of health behaviors and indicators such as physical activity, weight, diet, heart rate, blood pressure, and sleep. Analyzing this data could significantly change how we study human behavior and intervene to improve health. For instance, a large-scale study utilizing data from a diet-tracking app might reveal patterns and trends in dietary habits, which could inform public health initiatives or nutrition counseling strategies.
EHRs and large-scale data analysis
EHRs and large-scale data analysis have emerged as powerful tools for predicting patient outcomes and disease surveillance. For example, during pandemics, analyzing extensive healthcare data can help identify outbreak hotspots, monitor disease progression, and assess the effectiveness of interventions. This type of big data analytics played a significant role in managing the COVID-19 pandemic, where data from various sources was used to track the spread of the virus and develop mitigation strategies.
Moreover, EHRs provide a comprehensive patient history, which can be used to forecast possible health risks and patient outcomes. For instance, an AI-powered predictive model might analyze EHR data to identify patients at high risk of developing diabetes based on factors like age, body mass index (BMI), family history, and past health conditions. Such predictive analytics could enable earlier interventions and possibly prevent the onset of the disease.
Perspectives awaiting big data analytics in healthcare
Artificial intelligence (AI) and machine learning (ML) have shown immense promise in complementing big data analytics in healthcare. By training AI models on the extensive amounts of structured and unstructured data, healthcare providers can better predict patient outcomes, identify potential risks, and automate routine tasks. For example, AI can be used to analyze medical imaging data for early disease detection or to analyze patient medical history and genetic data to predict disease risk.
Enhancing disease outbreak prediction and management
Recent advances in big data analytics have shown its potential in predicting and managing disease outbreaks. By analyzing patterns from various data sources, including global health records, social media posts, climate data, and more, big data analytics can identify potential outbreaks before they occur, providing vital early warning signals.
For example, the Centers for Disease Control and Prevention (CDC) PulseNet is a significant example of big data analytics in action. PulseNet is a national laboratory network detecting bacterial enteric (intestinal) disease outbreaks. By performing DNA fingerprinting on bacteria using whole genome sequencing (WGS), PulseNet scientists can identify clusters of people sickened by bacteria with the same DNA fingerprint, which is a potential indicator of an outbreak.
Moreover, disease outbreak detection heavily relies on frontline vigilance, where healthcare workers need to maintain an elevated level of suspicion regarding unusual symptoms. Medical institutions worldwide need to ensure this information is consistently shared and updated, thereby improving the chances of identifying and mitigating disease outbreaks in their early stages.
Building an integrated and prepared global health system
With the lessons learned from the COVID-19 pandemic, the future of big data analytics in healthcare involves building a more prepared and resilient global health system. This involves enhancing the preparedness of healthcare providers and developing clear, well-drilled plans of action. It also includes securing access to vaccines and other essential medical supplies globally, as the world’s ability to discern and stop the next pandemic is only as good as its weakest link. This global vision can be facilitated by the effective use of big data analytics, ensuring that when the next pandemic inevitably emerges, healthcare professionals will be empowered to stop the spread earlier and limit its impacts.
As highlighted in the numerous research studies, it’s clear that big data analytics has the potential to revolutionize the healthcare sector by supporting more informed decision-making, fostering innovation, and enhancing care quality. As noted, the ability to process and analyze high-volume, high-velocity, and high-variety information not only paves the path for new care delivery models but also offers the promise of improved clinical decision support, disease surveillance, and population health management.
Concluding thoughts
Despite the immense promise and potential, it is also clear that the journey to realize the full benefits of big data analytics in healthcare is far from complete. Many hospitals and health organizations are still grappling with the challenges associated with developing and optimizing their big data analytics capabilities.
While there may be obstacles on the path toward maximizing the benefits of big data analytics in healthcare, the potential rewards greatly outweigh these challenges. As more healthcare providers recognize the immense value that big data analytics can bring, we can expect a shift towards more data-driven and evidence-based practices in the healthcare sector. The successful application of big data analytics in healthcare will not only lead to improved care outcomes and reduced costs but also support the broader transformation of the healthcare sector into a system that is responsive, efficient, and patient-centered. Indeed, the future of healthcare lies in leveraging the power of big data, and we are on the precipice of a new era in healthcare delivery. The journey may be challenging, but the destination is undoubtedly worth it.